Six artificial intelligence trends for engineers and scientists
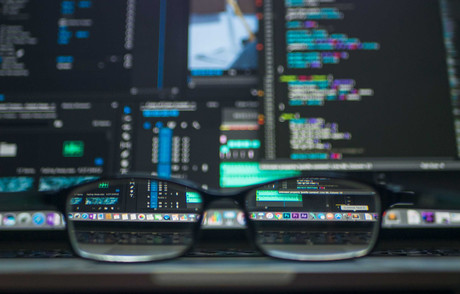
For those involved in researching, developing and launching AI applications there is still much learning and adapting to new concepts and skills to be done.
As AI, deep learning, data analytics, IoT and other concepts intersect, applications that once seemed futuristic come closer to reality. However, those tasked with researching, developing and launching these applications are still learning and adapting to new concepts and skills for their evolving job roles.
With AI becoming more prevalent across industries, there is a growing need to make it broadly available, accessible and applicable to engineers and scientists with varying specialisations. The complexity of larger datasets, cloud computing, embedded applications, and bigger development teams will drive solution providers towards interoperability, greater collaboration, reduced reliance on IT departments, and higher productivity workflows.
1. AI is not just for data scientists
Engineers and scientists, not just data scientists, will drive the experimentation and adoption of deep learning.
Technical curiosity, business imperatives to reap the promise of AI, and automation tools will empower more engineers and scientists to adopt AI. New workflow tools are simplifying and automating data synthesis, labelling, tuning, and deployment, thus making AI accessible beyond data scientists. These tools are also broadening the breadth of applications from image and computer vision to time-series data like audio, signal, and IoT that are common in numerous engineering domains. Example applications range from unmanned aerial vehicles (UAV) using AI for object detection in satellite imagery, to improved pathology diagnosis for early disease detection during cancer screenings.
2. Application and domain specialisation
Industrial applications are becoming a major consumer of AI but bring new demands for specialisation.
Smart cities, predictive maintenance, Industry 4.0, and other IoT and AI-led applications demand a set of criteria be met to become reality. For example, safety-critical applications that need increased reliability and verifiability; low-power, mass-produced and moving systems that require small form factors; and advanced mechatronics design approaches that integrate mechanical, electrical and other components.
3. Interoperability
Interoperability will be critical to assembling a complete AI solution.
The reality is, there isn’t a single framework that can provide ‘best-in-class’ for everything in AI. Currently, each deep learning framework tends to focus on a few applications and production platforms, while effective solutions require assembling pieces from several different workflows. This creates friction and reduces productivity. Organisations like ONNX.ai are focusing on addressing these interoperability challenges, which will enable developers to freely choose the best tool, more easily share their models, and deploy their solutions to a wider set of production platforms.
4. Cloud computing
Public clouds will increasingly be the host platform for AI, will evolve to reduce complexity, and will have reduced reliance on IT departments.
Powerful GPU instances, flexible storage options, and production-grade container technology are just a few of the reasons that AI applications are increasingly cloud-based. For engineers and scientists, cloud-based development eases collaboration and enables on-demand use of computing resources rather than buying expensive hardware with limited lifespan.
5. Edge computing
Edge computing will enable AI applications in scenarios where processing must be local.
Advances in sensors and low-power computing architectures will enable edge computing with high-performance, real-time, and increasingly complex AI solutions. Edge computing will be critical to safety in autonomous vehicles that need to understand their local environment and assess driving options in real-time. This has promise to yield huge cost savings for remote locations with limited or expensive Internet connectivity, like deep sea oil platforms.
6. Complexity necessitates greater collaboration
The increased use of machine learning and deep learning in complex systems will necessitate more and greater collaboration.
Data collection, synthesis and labelling are increasing the scope and complexity of deep learning projects, requiring larger and decentralised teams. Systems and embedded engineers will require flexibility to deploy inference models to data centres, cloud platforms, and embedded architectures such as FPGAs, ASICs, and microcontrollers. These teams will also need expertise in optimisation, power management and component reuse. Engineers at the centre of collaboration, developing deep learning models, will need tools to experiment with and manage the ever-growing volumes of training data and the lifecycle management of the inference models they handoff to system engineers.
To hear about how engineers are taking advantage of AI, register for MATLAB EXPO, touring Australia and New Zealand in May: https://www.matlabexpo.com/au/2019.html.
In a digital world, why is value still hard to find?
At all levels of an industrial system there are a number of barriers that make it difficult to...
Machine learning in manufacturing process control: How ARDI enhances operational efficiency
With increasing data availability, machine learning has become a powerful tool in the...
Verifying and Validating AI in Safety-Critical Systems
In the era of AI-enabled safety-critical systems, validation and verification is becoming crucial...